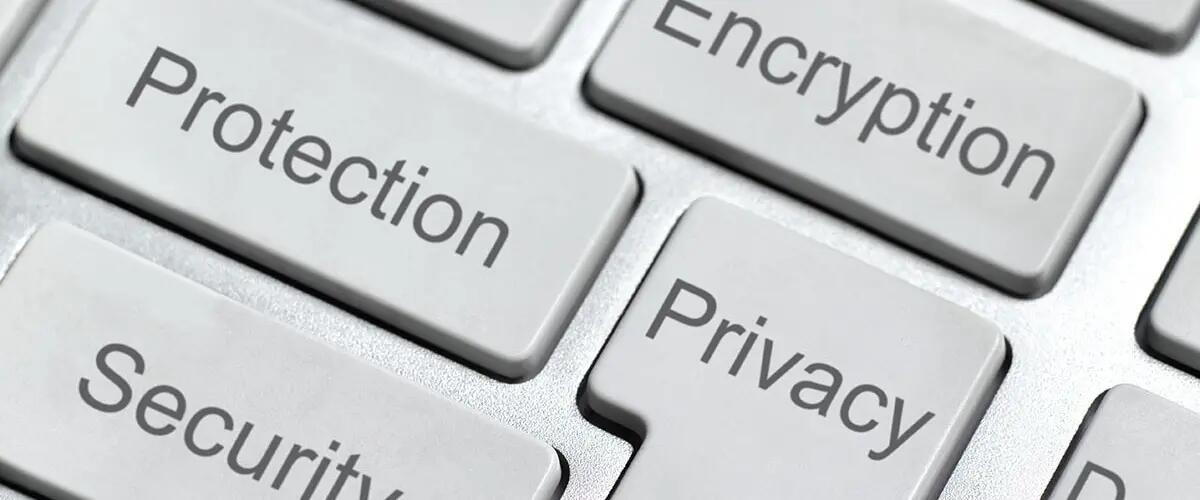
A professor's quest to protect data and make it more equitable
Jaideep Vaidya is a distinguished professor of Computer Information Systems and director of the Institute for Data Science, Learning and Applications housed within Rutgers Business School. His work focuses on data mining, data management, security, and privacy.
Like a data-mining sleuth, Professor Vaidya uncovers the problematic ways data is amassed and analyzed and he develops ways to better protect the privacy of individuals – of everyone, really – whose information is among the mega amounts of data stored in our modern world. Vaidya’s goal is to prevent information about our health, our DNA or even such everyday tasks as our driving from being misused. He is also busy creating new models so the detailed data being collected by hospitals, government agencies and companies leads to more accurate and equitable insights and ultimately, results in better practices, protections, and policies.
“The safe and responsible use of data is something that I care about,” Vaidya said. “That underlies all of the work that I’m doing.”
Vaidya was recently named a fellow of the American Association for the Advancement of Science in recognition of his significant contributions to the field of privacy protection in data analytics, information sharing and access control management. Among other honors for his pioneering work, Vaidya was named a fellow of the Institute of Electrical and Electronics Engineers in 2021.
Vaidya answered some questions below about his research:
Tell us about your latest research.
My current work focuses on examining the security and privacy challenges facing biomedical data repositories, and exploring how analytics can contribute to precision medicine. Ensuring the privacy and security of biomedical data is a significant challenge. The requirements for the protection of this sensitive data change depending on the situation and purpose for the access – for example, in an emergency, you would want the attending ER personnel to be able to access your medical records quickly though in non-emergency situations you may want to limit access. I’ve obtained funding from the federal government to develop new methodologies and innovative technologies to enable data sharing while respecting privacy and security considerations. Key results would include the development of techniques for synthetic (artificially generated) data generation, which will be a significant focus for the next phase of my career. This is crucial since high-fidelity synthetic data represents a pathway through which we can leverage the value from data while still protecting individually identifiable information. My team and I have another two-year grant from the National Science Foundation to enable the systematic study of synthetic data. Our work also further facilitates the training and development of new AI models and technologies since modern AI has a voracious appetite for data, and given the sensitivity of data, synthetic data will be primarily utilized instead of real data in the future.
I’m also working on the auditability and reproducibility of data analysis to better ensure fairness and equity. One of our new projects focuses on the verification of outsourced computation for genomic data. Along with collaborators from Case Western and University of Texas, we have just received a $3.2 million, 5-year grant from the National Libraries of Medicine and the National Human Genome Research Institute (part of the NIH) to get an accurate “preview” for the results of collaborative Genome-wide Association Studies in an efficient, verifiable, and privacy-preserving way. This will build on my work to reduce the computational burden for both clients and servers while ensuring integrity verification.
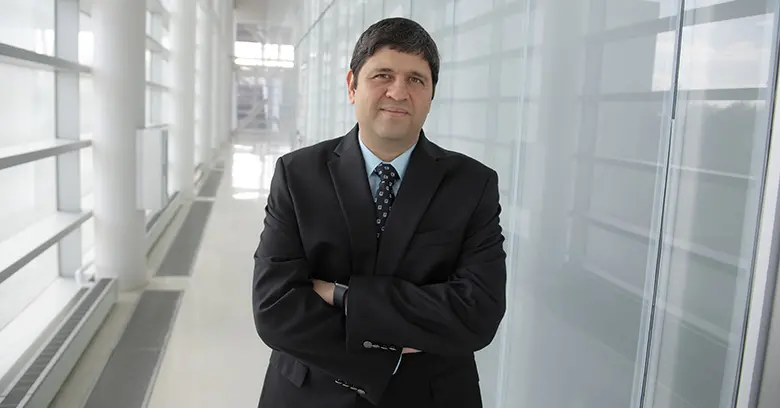
How does this build on your previous work?
I am expanding on my work in access control and security to enable dynamic configuration of access control policies and low-cost enforcement of access control in edge computing environments. With a complex system consisting of multiple underlying systems, participants, and evolving environmental constraints, it is important to provide auditability to reduce the possibility of misuse (gamification) and increase user confidence. One possibility is to use a blockchain-based decentralized approach that essentially records each decision along with an underlying justification including the provided input and interaction between the different collaborators. By coupling it with a game-theoretic approach, it is possible to minimize the cost of auditing while making the overall approach incentive compatible.
After studying computer engineering and computer science as a student, what led you down the road of data mining and security?
Initially, I was interested in pure computer science, things like networking and operating systems, but then I realized that data had a story to tell. That was right around the time 9-11 occurred and all of a sudden security became very important. The biggest question at the time was if this was a dichotomy where you had to choose between privacy and security. There was some economics research which said it wasn’t mutually exclusive, but they had not applied it to the field of data analytics. That’s where my research really started. We have all of this data. We want to get value out of it, but can we make sure we protect the privacy of the information as well.
Tell us about the role of the Institute for Data Science, Learning and Applications and some of the work that’s been done there.
The institute has three primary focuses, carrying out research, looking at potential educational activities, and providing expertise in data science to the campus and community. We have work that spans security, privacy, and data analytics management in different domains. One of the newer efforts the Institute is leading is in health equity. We’re looking at data and trying to make sure new machine learning models don’t magnify inequities. We’ve seen two kinds of bias, some systematic bias and second, the machine learning is very much driven by the data used to build the models. We may not have sufficiently diverse data so the model itself is biased. The institute is working on this with the School of Communications and Information and the School of Public Health in Newark. While the problems of health equity are broad, we’re focusing on mental and behavioral health. We know there are huge inequities there. For example, if you’re looking at the diagnosis of schizophrenia, there is bias and there are problems in the way things are recorded and in certain cases, there is stigma associated with certain words. The collaborators we’re working with have expertise so they can see how it’s problematic. We want to solve this by coming up with trustworthy models.
- Susan Todd
Press: For all media inquiries see our Media Kit